
欢迎加群讨论技术,1群:677373950(满了,可以加,但通过不了),2群:656732739
评价
排名
6
文章
6
粉丝
16
评论
8
{{item.articleTitle}}
{{item.blogName}} : {{item.content}}
ICP备案 :渝ICP备18016597号-1
网站信息:2018-2025TNBLOG.NET
技术交流:群号656732739
联系我们:contact@tnblog.net
公网安备:
50010702506256

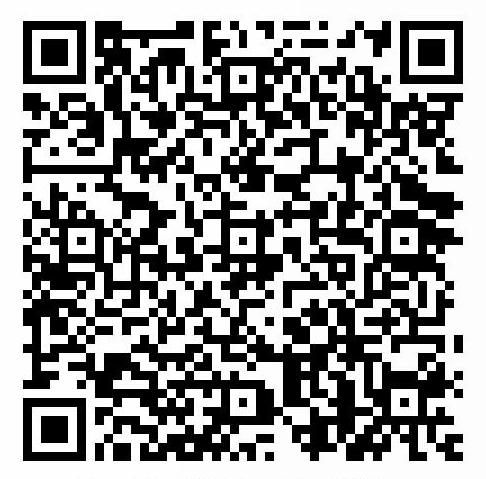
欢迎加群交流技术